Sample Driver License Knowledge Tests ASL Sample Test 1 ASL Sample Test 1 About. The History of the Department of Motor Vehicles; Department of Motor Vehicles. Neuvoo™ 【 1 021 307 Asl Residential Driver Job Opportunities in USA 】 We’ll help you find USA’s best Asl Residential Driver jobs and we include related job information like salaries & taxes. It’s quick and easy to apply online for any of the 1 021 307 featured Asl Residential Driver jobs.
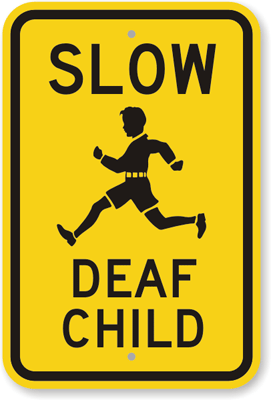
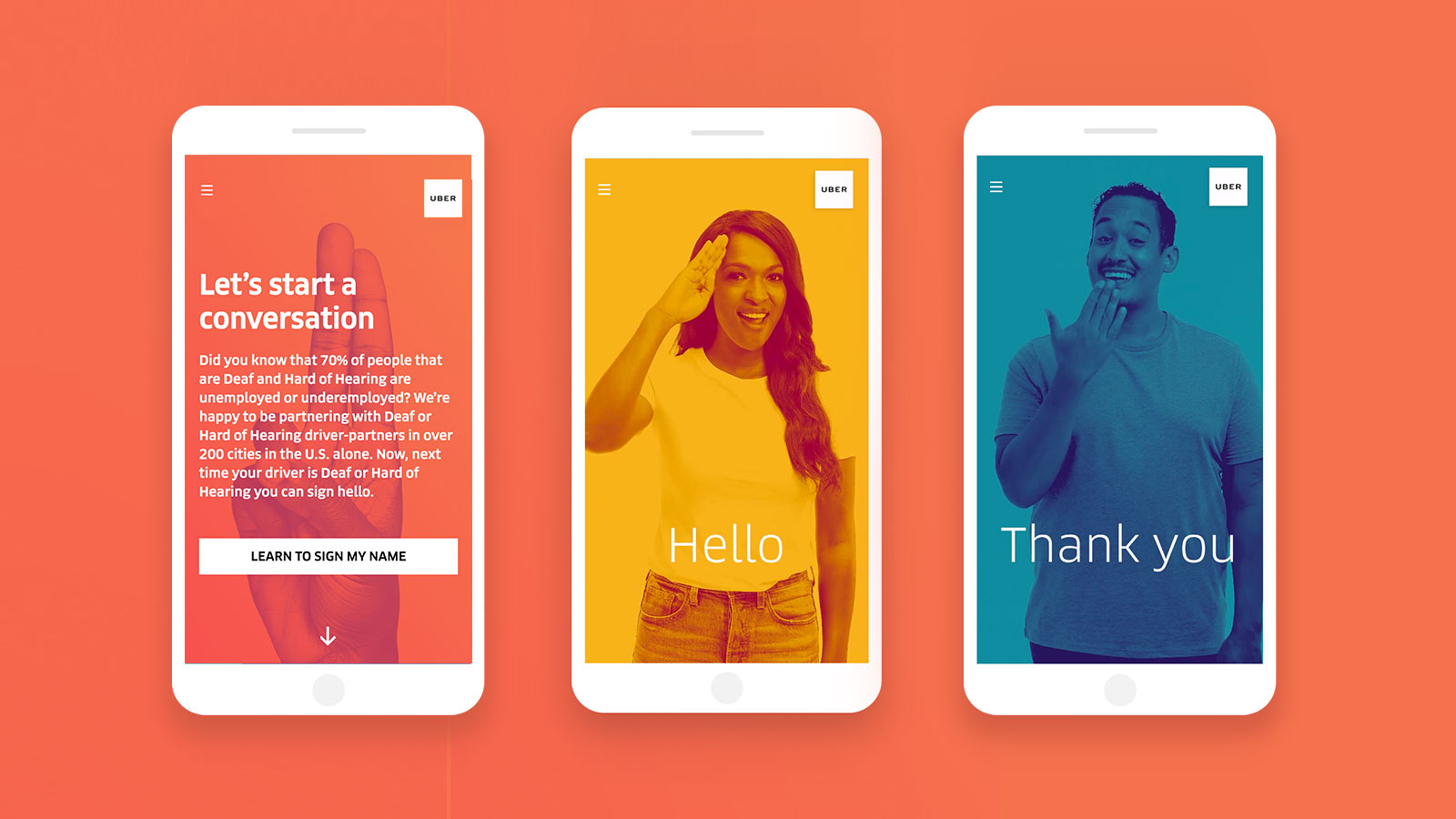
Every brigade combat team (BCT) and support brigade in the Army has a mobile minidistribution center that stocks repair parts and perhaps also class II (clothing and individual equipment), IIIP (packaged petroleum, oils, and lubricants), and IV (construction and barrier materials) items with national stock numbers (NSNs) in its authorized stockage list (ASL). This minidistribution center, called the supply support activity (SSA), is the key to high equipment readiness. When equipment fails and becomes not mission capable (NMC), but the needed parts are on hand in the SSA, that equipment can be returned to action very quickly. However, when the parts are not available in the supporting SSA, it can sometimes take awhile to get them, which only delays returning equipment to a mission capable status.
In operations in Iraq, getting the part from the United States by air (if the part is well positioned for quick shipment) or from theater stocks in Kuwait takes an average of a little more than 10 days, with some shipments taking longer. If the item is in short supply at U.S. distribution centers, the wait can be much longer. Not having parts in the ASL sometimes leads units to take extraordinary actions, such as controlled exchanges, to get equipment back on line rather than accept lengthy downtimes on critical end items.
Studies by RAND Arroyo Center have confirmed that high-performing ASLs have the greatest direct affect on equipment readiness through their impact on supply chain processes and resources. (Reliability, of course, is the other central factor affecting equipment readiness.) For example, a RAND Arroyo study at the National Training Center at Fort Irwin, California found that a 10-point swing in the ASL fill rate changed the equipment readiness rate by 4 percentage points. However, Army ASL fill rates were often under 20 percent in the late 1990s because of very limited breadths of parts and some issues concerning how depths were computed. (“Breadth” refers to the number of different parts stocked. “Depth” refers to the number of each part stocked.)
Development of Dollar Cost Banding
To address the low ASL fill rates, RAND Arroyo developed Dollar Cost Banding (DCB). Piloted in 1998, DCB introduced three things to ASL management: tying the decision of what and how much to stock to both the benefits produced and the resources required, emphasizing the need to deal with highly variable demand, and using automated exclusion criteria.
The hypothesis underlying DCB development was that, even if the benefit of adding a part is relatively low, if the part’s cost and size are minimal and its absence can affect readiness, it may be worth stocking. The DCB concept was introduced using heuristics that adjusted add-and-retain thresholds in terms of the number of demands based on item cost (inventory investment) and size (storage space). Basically, the DCB rules said that the smaller and less expensive an item is, and if it is coded essential or has had high-priority demands, the threshold for stocking it should be lessened. The add-and-retain criteria for big, expensive items that are often critical to readiness were not changed. This change increased the breadth of ASLs by adding more of the small, inexpensive items that are often needed in conjunction with the more expensive items to correct NMC or deadlining faults.
The second, and less well known, aspect of DCB was a complete change in how inventory depth is computed. Inventory levels with DCB are set by using iterative simulations of the demand streams at the national item identification number (NIIN) level to achieve customer-wait-time goals that vary based on the investment and storage resources associated with the NIIN. This contrasts greatly with the former “days of supply” method, which used only the mean demand rate and thus did not compensate for the variability or timing of demands. (Demands during peak training periods were averaged or were smoothed out with zero or low demands during periods in garrison.)
The third aspect of DCB was a set of parameters that automatically exclude certain NIINs that are not desirable to stock in SSAs in order to reduce the ASL review workload. Examples of the criteria used are acquisition advice code, nomenclature, class of supply, and Federal supply class. The parameters have been continually updated based on feedback from the field. The central idea behind exclusions was to keep items from being recommended for ASL stockage that were not critical to warfighting or that the unit could otherwise wait to obtain through an order-ship cycle.
Initially, DCB was implemented by having a central team at RAND develop the ASL recommendations. The central team also assisted in reconfiguring warehouse storage and participated in the SSAs’ review of the recommendations. After successful pilots, this led to the incorporation of DCB into Army policy in 2000 and a rapid, successful rollout through about half the SSAs in the Army. Fill rates increased by about 10 percent in those SSAs that adopted DCB. In 2001, DCB became available in the Integrated Logistics Analysis Program (ILAP), which allowed SSAs to initiate and run their own ASL reviews. However, without the role played by the central team, the results became less consistent as inventory expertise varied among SSAs and other demands on personnel time sometimes impeded effective implementation of the recommendations.
Introduction of Enhanced DCB
At about this time, RAND Arroyo Center developed the Equipment Downtime Analyzer (EDA), which also was added as a module within ILAP. The EDA archives daily NMC equipment reports. This information is very valuable because it identifies all of the parts ordered to return a system to mission capable status. With EDA data, it is now possible to develop a critical parts list of those parts that consistently deadline Army equipment.
The EDA critical parts list was used to develop Enhanced DCB (EDCB), which, initially, simply changed the criticality criteria. In DCB, a part was considered “critical” based on the essentiality code or the use of high-priority requisitions for the part. The problem was that, with these rules, most parts (85 percent) are deemed “critical.” Using the EDA critical parts list, we have found that the list of true readiness drivers is much narrower—now only 35 percent of demanded items. Therefore, EDCB allows us to concentrate the allocation of limited SSA storage capacity and inventory investment on these more critical parts.
EDCB was piloted with two BCTs at Fort Riley, Kansas, in 2002. This pilot targeted three key systems—the M1A1 Abrams tank, the M88A1 recovery vehicle, and the M9 armored combat earthmover—with great success. The readiness-driver fill rates for targeted systems improved considerably. Consequently, awaiting parts time, and, thus, overall time for deadlining repairs, fell dramatically, thereby increasing readiness for each BCT.
Impact of Operation Iraqi Freedom
Before implementation of EDCB could be expanded, Operation Iraqi Freedom (OIF) began. This put further rollout of EDCB on hold as units that were to prototype the new algorithm turned their attention to deployment preparations and then combat operations. When units initially deployed to OIF, they generally took the ASLs they had at home with little change (or they fell in on ASLs from Army pre-positioned stocks). Units that had been involved in the DCB rollout found that their home-station ASLs were relatively effective, at least initially. For most units, the breadth of parts demanded in OIF was similar to what they experienced when training at home station, so accommodation rates held up.
However, a lack of connectivity with Standard Army Management Information Systems (STAMIS), combined with severe distribution challenges in 2003, significantly hampered replenishment and quickly depleted those deployed ASLs. Without reliable replenishment, ASL satisfaction rates fell to less than 10 percent, making the ASLs ineffective. Another factor hampering the recovery of satisfaction rates was that depth in the home-station ASLs that units deployed with had been calculated using the actual replenishment times for each NIIN at home station (a minimum of 10 days was enforced); but those replenishment times had not yet been achieved in OIF.
Realizing the need to better match ASLs with growing demands, theater logisticians began ASL reviews using DCB (in ILAP), which did lead to improvements in depth by using actual replenishment lead times and demand rates for OIF. Combined with improved distribution, these enabled ASLs to recover to about 30-percent fill rates. However, additional problems hampered the effectiveness of ASL reviews: Deployed demand histories were limited in duration to less than the 2 years used in DCB; as rotations began, many units were task organized, and so deployed SSAs were used to support different types of units; and equipment changes sometimes occurred (such as the addition of up-armored high-mobility, multipurpose, wheeled vehicles). All of these factors rendered the use of deployed SSA demand histories only partially effective for forecasting future demands and setting inventory levels.
To tackle these issues, RAND began to assist in building virtual demand histories for units. These histories were based on moving unit demand streams at the company level, making adjustments to account for limited demand histories, and using the demand histories of proxy units to model requirements for equipment new to a unit. Using these demand streams, EDCB was applied, with recommendations passed to SSAs in Iraq, Kuwait, and Afghanistan.
Between late 2004 and the middle of 2006, acceptance and implementation of the recommendations was mixed. Those SSAs that implemented EDCB experienced dramatic ASL improvements; those that did not saw stagnant performance. This led to 20- to 30-point gaps in fill rates among SSAs supporting similar units. ASL reviews also often took a long time, which impeded performance improvement during a significant portion of year-long rotations. However, where the recommendations were implemented, readiness-driver fill rates climbed significantly above those of non-readiness drivers. Despite variations by SSAs in the adoption of EDCB recommendations, the overall readiness-driver fill rate for OIF had climbed to almost 50 percent by late 2006.
One issue that sometimes created delays was the sheer time needed to review the thousands of recommendations that came from DCB and EDCB. This problem was aggravated if the recommendations did not fit within the existing storage configuration of an SSA. Another challenge was that the large numbers of ASL changes required to implement the recommended “adds,” “deletes,” and requisition objective (RO) changes created a greater workload than some SSAs could handle given their daily ongoing work.

|
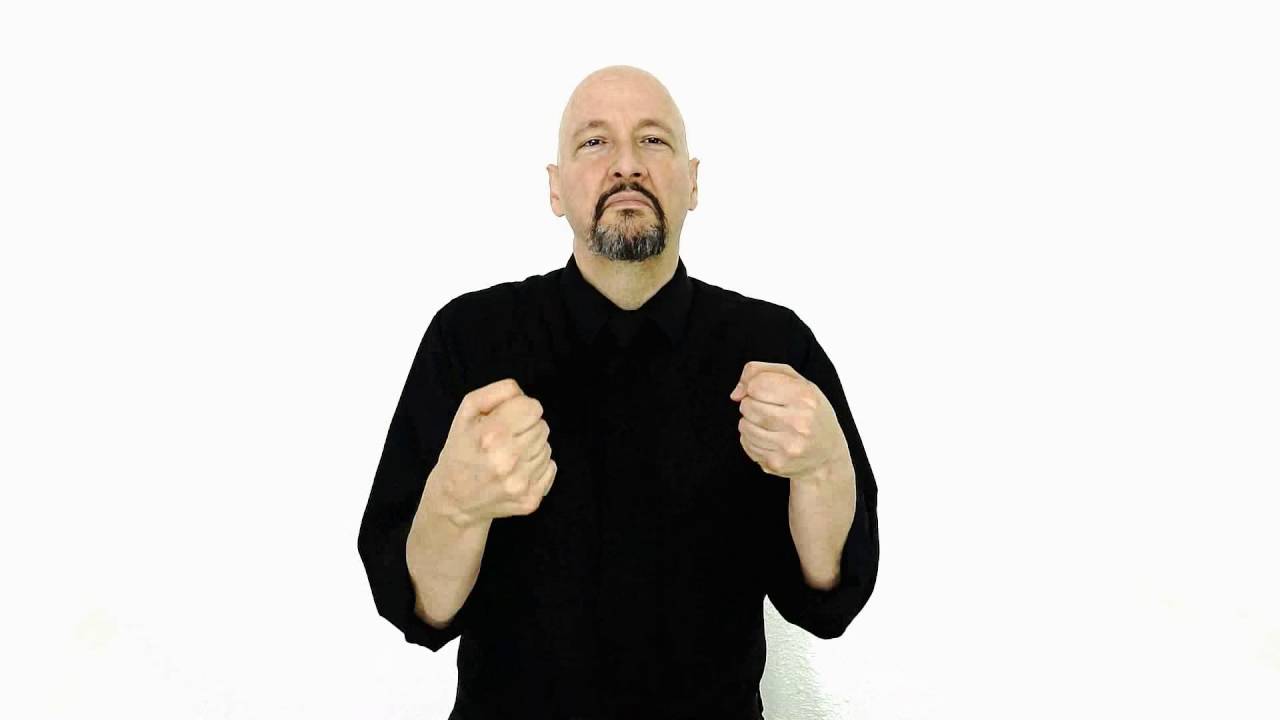
Enhancement of ASL Management With IROC
Based on these OIF issues, and other lessons learned from this body of ASL research, RAND Arroyo Center has developed a new model for computing ASL recommendations called the Inventory Readiness Optimizer with Constraints (IROC).
IROC is essentially a product improvement of the EDCB algorithm. It is based on a mixed-integer programming formulation that is intended to optimize the readiness contribution of the ASL, subject to constraints on inventory investment, transition workload, and the number and volume of storage locations by type (such as small bin, medium bin, shelf, rack, and bulk). A weighting derived from the EDA database indicates the relative criticality of parts, rather than simply considering parts as critical or not. The recommendations from this process were then input into a simulation to determine the resulting readiness (down days) and to establish curves of ASL performance and readiness impacts versus resources.
IROC was prototyped among units undergoing modularity transformation and led to many insights on how to overcome the OIF ASL issues (particularly storage feasibility and transition workload issues) affecting the implementation of ASL recommendations.
RAND Arroyo Center uses IROC results to fine-tune EDCB and improve the recommendations provided to deployed units by—
Please find your product drivers & manuals using the search box on the left side of this page. You can download the drivers under the Support & Download tab of the product page.
Drivers Asleep While Driving
If you cannot find your product on this website, please check ourLegacy Products Database.
All other inquiries such as troubleshooting hardware issues or assistance on how to use a product please call (800) 503-2378.
Drivers Falling Asleep At The Wheel
You can also submit tickets using our Support Ticket Center.
Drivers Licence Asl
Notice: To ensure you have the latest drivers for your Diamond AMD Graphics card, download the drivers from the following link below: http://support.amd.com/en-us/download
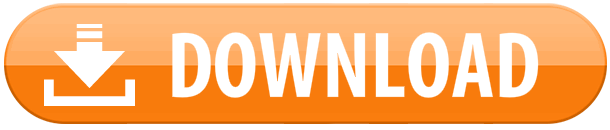